Harnessing Machine Learning to Combat Spam: A Guide for Your Business
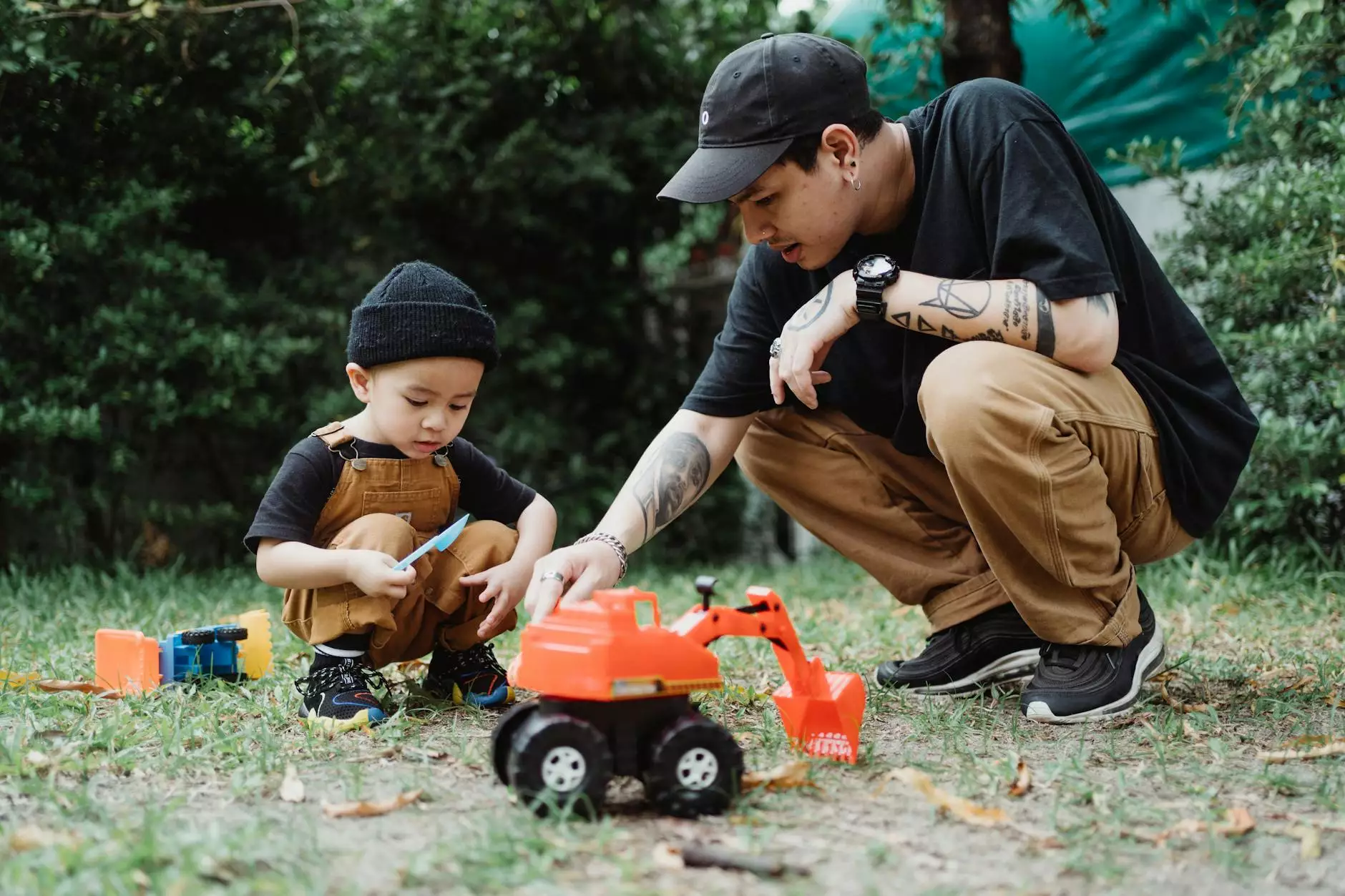
In today's digital landscape, spam has become an omnipresent challenge for businesses. With unsolicited messages flooding our inboxes and disrupting our daily operations, organizations must adopt advanced strategies to manage and mitigate these nuisances. This is where machine learning comes into play, revolutionizing how we address spam. At Spambrella.com, we recognize the significance of leveraging sophisticated technologies to enhance IT services and security systems. This article delves into how machine learning can help transform your approach to spam.
Understanding Machine Learning
Machine learning is a subset of artificial intelligence (AI) that empowers computer systems to learn and improve from experience without being explicitly programmed. This capability is particularly valuable in the realm of spam detection, where traditional rules-based approaches often fall short.
By analyzing vast amounts of data, machine learning algorithms can identify patterns and make predictions based on that data. This process involves several stages:
- Data Collection: Gathering data from various sources, including emails, user interactions, and past spam incidents.
- Data Preprocessing: Cleaning and formatting data to ensure it's suitable for analysis.
- Training: Using labeled datasets to train machine learning models, allowing them to learn the characteristics of spam versus legitimate messages.
- Testing and Validation: Evaluating the accuracy of the model to ensure it can effectively differentiate between spam and non-spam.
- Deployment: Integrating the model into existing email systems to automate spam detection and response.
The Impact of Spam on Businesses
The implications of spam on business operations can be significant. Spam not only clutters inboxes but also poses serious risks, such as:
- Loss of Productivity: Employees waste precious time sifting through spam, diverting attention from more critical tasks.
- Security Threats: Spam emails often serve as vehicles for phishing attacks, malware, and other cybersecurity threats.
- Reputational Damage: Excessive spam can tarnish a business's reputation, leading to decreased trust from customers and partners.
Machine Learning and Spam Detection
Incorporating machine learning into your spam detection strategy offers an effective solution to combat these challenges. Here’s how it works:
1. Anomaly Detection
Machine learning models can identify anomalies in communication patterns, flagging messages that deviate from typical behavior. For example, if an email from a known contact suddenly contains links to unfamiliar websites, the model can recognize this as a potential threat.
2. Natural Language Processing
Natural language processing (NLP) is a branch of machine learning that focuses on the interaction between computers and humans through language. By analyzing the content of emails, NLP algorithms can detect spammy language and phrases commonly associated with unsolicited messages.
3. Continuous Learning
One of the standout features of machine learning is its ability to learn continuously. As new spam tactics emerge, your machine learning model can adapt by updating its knowledge base with new examples of spam and non-spam messages.
4. User Feedback Integration
Incorporating user feedback into the machine learning process can enhance the accuracy of spam detection. Users marking messages as spam or not spam provide valuable data for refining the model's algorithms and improving its predictive capabilities.
Real-World Applications of Machine Learning in Spam Management
Many businesses have successfully implemented machine learning to combat spam. Here are some notable applications:
Email Filtering
Organizations can use machine learning algorithms to filter out spam emails before reaching employees’ inboxes. Solutions like Google's Gmail rely heavily on machine learning to categorize and prioritize email, effectively reducing spam.
Security Monitoring
Machine learning can enhance security systems by monitoring communication channels and identifying potential spam or phishing attacks in real-time. This proactive approach helps in safeguarding sensitive data.
Customer Engagement
By utilizing machine learning to analyze customer behaviors and preferences, businesses can tailor their messaging to avoid being classified as spam, ensuring better engagement rates while maintaining compliance with communication regulations.
Best Practices for Implementing Machine Learning Spam Solutions
To effectively implement machine learning in managing spam, consider the following best practices:
- Invest in Quality Data: Ensure that you have access to high-quality, relevant datasets for training your machine learning models.
- Choose the Right Algorithms: Select algorithms that best fit your specific spam detection needs; common choices include decision trees, support vector machines, and neural networks.
- Monitor and Update: Continuously monitor the performance of your machine learning models and update them with new data to maintain effectiveness.
- Educate Employees: Provide training for employees on identifying spam and phishing attempts, and encourage them to report suspicious emails.
Conclusion
The integration of machine learning into business operations represents a transformative approach to combating spam. By leveraging advanced algorithms and analytical techniques, companies can streamline their communication, enhance security, and boost productivity. At Spambrella.com, we are committed to empowering businesses through innovative IT services and security systems that embrace machine learning principles. Harness the power of advanced technologies to ensure your business remains free from the clutches of spam, allowing you to focus on what truly matters.